Spatial Vector-Based Approach to the ERP Analysis as Applied to an EEG-based Discrimination of Traffic Light Signals
DOI:
https://doi.org/10.15540/nr.6.4.170Keywords:
EEG spatial vectors, Traffic light perception, VEP, Discrimination value, Modified knn classifierAbstract
The purpose of the study was to assess the utility of the spatial vector-based representation of multichannel electroencephalography (EEG; when each spatial vector denotes an “instantaneous” sample of cortical activation evolving over time) in the analysis of cortical responses to visual stimulation—as opposed to the traditional, temporal vector-based approach, when vectors are associated with distinct EEG channels. This representation was used in the analysis of EEG collected in the virtual traffic light environment with the attempt to determine the color of traffic light perceived by four participants. Kruskal-Wallis (K-W) analysis of variance was implemented for selected EEG electrodes. To utilize all available information, discrimination value was evaluated next for 32-dimensional EEG spatial vectors followed by modified “k nearest neighbors” (knn) classification. K-W test indicated that EEG samples at selected electrodes are different between different colors of traffic light and when observed for specific latencies. The average accuracy of a modified three-class knn classifier was approaching 60% (the random assignment would yield approximately 33%) for the specific poststimuli latencies. The proposed technique allows analyzing stimulation-synchronized cortical activity with the temporal resolution generally determined by the sampling rate of the neuroimaging modality. The discrimination value appears instrumental for predicting the clusterability of data assessed. Stimulation-evoked cortical responses are often of interest in studies of human cognition. The proposed technique may overcome the low signal-to-noise limitation of the traditional evoked response potential (ERP) analysis and possibly provide means to assess such responses under the real-time constraint.
References
Bayliss, J. D., & Ballard, D. H. (1998). The effects of eye tracking in a VR helmet on EEG recording. Technical Report 685, University of Rochester National Resource Laboratory for the Study of Brain and Behavior.
Bayliss, J. D., & Ballard, D. H. (2000). A virtual reality testbed for brain–computer interface research. IEEE Transactions on Rehabilitation Engineering, 8(2), 188–190. https://doi.org/10.1109/86.847811
Clark, V. P., & Hillyard, S. A. (1996). Spatial selective attention affects early extrastriate but not striate components of the visual evoked potentials. Journal of Cognitive Neuroscience, 8(5), 387–402. https://doi.org/10.1162/jocn.1996.8.5.387
Duda, R. O., Hart, P. E., & Stork, D. G. (2000). Pattern Classification, 2nd edition. New York, NY: Wiley.
Heslenfeld, D. J., Kenemans, J. L., Kok, A., & Molenaar, P. C. M. (1997). Feature processing and attention in the human visual system: An overview. Biological Psychology, 45(1–3), 183–215. https://doi.org/10.1016/S0301-0511(96)05228-3
Holcomb, P. J., & McPherson, W. B. (1994). Event-related brain potentials reflect semantic priming in an object decision task. Brain and Cognition, 24(2), 259–276. https://doi.org/10.1006/brcg.1994.1014
Hoque, M. R. U., & Tcheslavski, G. V. (2018). Can electroencephalography improve road safety? An EEG-based study of driver's perception of traffic light signals in a virtual environment. International Journal of Vehicle Safety, 10(1), 78–86. https://doi.org/10.1504/IJVS.2018.093062
Kenemans, J. L., Kok, A., & Smulders, F. T. Y. (1993). Event-related potentials to conjunctions of spatial frequency and orientation as a function of stimulus parameters and response requirements. Electroencephalography and Clinical Neurophysiology, 88(1), 51–63. https://doi.org/10.1016/0168-5597(93)90028-N
Khaliliardali, Z., Chavarriaga, R., Gheorghe, L. A., & Millán, J. d. R. (2012). Detection of anticipatory brain potentials during car driving. 2012 Annual International Conference of the IEEE Engineering in Medicine and Biology Society, 3829–3832. https://doi.org/10.1109/EMBC.2012.6346802
Krauss, P., Metzner, C., Schilling, A., Tziridis, K., Traxdorf, M., Wollbrink, A., … Schulze, H. (2018). A statistical method for analyzing and comparing spatiotemporal cortical activation patterns. Scientific Reports, 8(1), 5433. https://doi.org/10.1038/s41598-018-23765-w
Kutas, M., & Hillyard, S. A. (1980). Reading senseless sentences: Brain potentials reflect semantic incongruity. Science, 207(4427), 203–205. https://doi.org/10.1126/science.7350657
Kutas, M., & Hillyard, S. A. (1984). Brain potentials during reading reflect word expectancy and semantic association. Nature, 307, 161–163. https://doi.org/10.1038/307161a0
Liang, S.-F., Lin, C.-T., Wu, R.-C., Huang, T.-Y., & Chao, W.-H. (2005). Classification of driver's cognitive responses from EEG analysis. 2005 IEEE International Symposium on Circuits and Systems, 1, 156–159. https://doi.org/10.1109/ISCAS.2005.1464548
Lin, C.-T., Chung, I-F., Ko, L.-W., Chen, Y.-C., Liang, S.-F., & Duann, J.-R. (2007). EEG-based assessment of driver cognitive responses in a dynamic virtual-reality driving environment. IEEE Transactions on Biomedical Engineering, 54(7), 1349–1352. https://doi.org/10.1109/TBME.2007.891164
Lin, C.-T., Lin, K.-L., Ko, L.-W., Liang, S.-F., Kuo, B.-C., & Chung, I.-F. (2008). Nonparametric single-trial EEG feature extraction and classification of driver’s cognitive responses. EURASIP Journal of Advances in Signal Processing, 2008, 849040. https://doi.org/10.1155/2008/849040
Ludwig, K. A., Miriani, R. M., Langhals, N. B., Joseph, M. D., Anderson, D. J., & Kipke, D. R. (2009). Using a common average reference to improve cortical neuron recordings from microelectrode arrays. Journal of Neurophysiology, 101(3), 1679–1689. https://doi.org/10.1152/jn.90989.2008
Mangun, G. R., Hillyard, S. A., & Luck, S. J. (1993). Electrocortical substrates of visual selective attention. In D. Meyer & S. Kornblum (Eds.), Attention and Performance XIV (pp. 219–243). Cambridge, MA: MIT Press.
Mourino, J., del R. Millan, J., Cincotti, F., Chiappa, S., Jane, R., & Babiloni, F. (2001). Spatial filtering in the training process of the brain domputer interface. 2001 Conference Proceedings of the 23rd Annual International Conference of the IEEE Engineering in Medicine and Biology Society (EMBS), 639–642. https://doi.org/10.1109/IEMBS.2001.1019016
Phyu, T. N. (2009, March). Survey of Classification Techniques in Data Mining. Proceedings of the International MultiConference of Engineers and Computer Scientists, Vol I. IMECS 2009, Hong Kong.
Rutiku, R., Aru, J., & Bachmann, T. (2016). General markers of conscious visual perception and their timing. Frontiers in Human Neuroscience, 10, 23. https://doi.org/10.3389/fnhum.2016.00023
Sherlin, L. H., Arns, M., Lubar, J., Heinrich, H., Kerson, C., Strehl, U., & Sterman, M. B. (2011). Neurofeedback and basic learning theory: Implications for research and practice. Journal of Neurotherapy, 15(4), 292–304. https://doi.org/10.1080/10874208.2011.623089
Strehl, U., Aggensteiner, P., Wachtlin, D., Brandeis, D., Albrecht, B., Arana, M., ... Holtmann, M. (2017). Neurofeedback of slow cortical potentials in children with attention-deficit/hyperactivity disorder: a multicenter randomized trial controlling for unspecific effects. Frontiers in Human Neuroscience, 11, 135. https://doi.org/10.3389/fnhum.2017.00135
Thorpe, S., Fize, D., & Marlot, C. (1996). Speed of processing in the human visual system. Nature, 381, 520–522. https://doi.org/10.1038/381520a0
Van Elk, M., van Schie, H. T., & Bekkering, H. (2010). The N400-concreteness effect reflects the retrieval of semantic information during the preparation of meaningful actions. Biological Psychology 85(1), 134–142. https://doi.org/10.1016/j.biopsycho.2010.06.004
Walsh, P., Kane, N., & Butler, S. (2005). The clinical role of evoked potentials. Journal of Neurology, Neurosurgery & Psychiatry, 76(Suppl. 2), ii16–ii22. https://doi.org/10.1136/jnnp.2005.068130
Wang, H., & Zhang, N. (2010, June). The analysis on vehicle color evoked EEG based on ERP method. Poster presented at the. 2010 4th International Conference on Bioinformatics and Biomedical Engineering (iCBBE), Chengdu, China.
Yeh, Y.-Y., Lee, D.-S., & Ko, Y.-H. (2013). Color combination and exposure time on legibility and EEG response of icon presented on visual display terminal. Displays, 34(1), 33–38. https://doi.org/10.1016/j.displa.2012.11.007
Yigal, A., & Sekuler, R. (2007). Interactions between working memory and visual perception: An ERP/EEG study. NeuroImage, 36(3), 933–942. https://doi.org/10.1016/j.neuroimage.2007.04.014
Downloads
Published
Issue
Section
License
Authors who publish with this journal agree to the following terms:- Authors retain copyright and grant the journal right of first publication with the work simultaneously licensed under a Creative Commons Attribution License (CC-BY) that allows others to share the work with an acknowledgement of the work's authorship and initial publication in this journal.
- Authors are able to enter into separate, additional contractual arrangements for the non-exclusive distribution of the journal's published version of the work (e.g., post it to an institutional repository or publish it in a book), with an acknowledgement of its initial publication in this journal.
- Authors are permitted and encouraged to post their work online (e.g., in institutional repositories or on their website) prior to and during the submission process, as it can lead to productive exchanges, as well as earlier and greater citation of published work (See The Effect of Open Access).
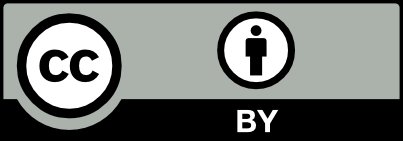