fMRI-EEG Fingerprint Regression Model for Motor Cortex
DOI:
https://doi.org/10.15540/nr.8.3.162Keywords:
EEG, fMRI BOLD, regression model, motor cortex, strokeAbstract
The combination of modern machine learning and traditional statistical methods allows the construction of individual regression models for predicting the blood oxygenation level dependent (BOLD) signal of a selected region-of-interest within the brain using EEG signal. Among the many different models for motor cortex, we chose the EEG Fingerprint one-electrode approach, based on rigid regression model with Stockwell EEG signal transformation, used before only for the amygdala. In this study we demonstrate the way of finding suitable model parameters for the cases of BOLD signal reconstruction for five individuals: three of them were healthy, and two were after a hemorrhagic stroke with varying degrees of damage according to Medical Research Council (MRC) Weakness Scale. The principal possibility of BOLD restoring using regressor model was demonstrated for all the cases considered above. The results of direct and indirect comparisons of BOLD signal reconstruction at the motor region for healthy participants and for patients who suffered from a stroke are presented.
References
Beckmann, C. F., DeLuca, M., Devlin, J. T., & Smith, S. M. (2005). Investigations into resting-state connectivity using independent component analysis. Philosophical Transactions of the Royal Society of London. Series B, Biological Sciences, 360(1457), 1001–1013. https://doi.org/10.1098/rstb.2005.1634
Bergstra, J., Yamins, D., & Cox, D. D. (2013). Making a science of model search: Hyperparameter optimization in hundreds of dimensions for vision architectures. Proceedings of the 30th International Conference on Machine Learning (ICML 2013), 28, 115–123.
Chang, C.-Y., Hsu, S.-H., Pion-Tonachini, L., & Jung, T.-P. (2018). Evaluation of artifact subspace reconstruction for automatic EEG artifact removal. 2018 40th Annual International Conference of the IEEE Engineering in Medicine and Biology Society (EMBC), 1242–1245. https://doi.org/10.1109/EMBC.2018.8512547
Custo, A., Vulliemoz, S., Grouiller, F., Van De Ville, D., & Michel, C. (2014). EEG source imaging of brain states using spatiotemporal regression. NeuroImage, 96, 106–116. https://doi.org/10.1016/j.neuroimage.2014.04.002
de Munck, J. C., Gonçalves, S. I., Mammoliti, R., Heethaar, R. M., & Lopes da Silva, F. H. (2009). Interactions between different EEG frequency bands and their effect on alpha-fMRI correlations. NeuroImage, 47(1), 69–76. https://doi.org/10.1016/j.neuroimage.2009.04.029
Delorme, A., & Makeig, S. (2004). EEGLAB: An open source toolbox for analysis of single-trial EEG dynamics including independent component analysis. Journal of Neuroscience Methods, 134(1), 9–21. https://doi.org/10.1016/j.jneumeth.2003.10.009
Emmert, K., Kopel, R., Sulzer, J., Brühl, A. B., Berman, B. D., Linden, D., Horovitz, S. G., Breimhorst, M., Caria, A., Frank, S., Johnston, S., Long, Z., Paret, C., Robineau, F., Veit, R., Bartsch, A., Beckmann, C. F., Van De Ville, D., & Haller, S. (2016). Meta-analysis of real-time fMRI neurofeedback studies using individual participant data: How is brain regulation mediated? NeuroImage, 124(Part A), 806–812. https://doi.org/10.1016/j.neuroimage.2015.09.042
Goense, J. B. M., & Logothetis, N. K. (2008). Neurophysiology of the BOLD fMRI signal in awake monkeys. Current Biology, 18(9), 631–640. https://doi.org/10.1016/j.cub.2008.03.054
Goldman, R. I., Stern, J. M., Engel, J., Jr., & Cohen, M. S. (2002). Simultaneous EEG and fMRI of the alpha rhythm. NeuroReport, 13(18), 2487–2492. https://doi.org/10.1097/01.wnr.0000047685.08940.d0
Gramfort, A., Luessi, M., Larson, E., Engemann, D. A., Strohmeier, D., Brodbeck, C., Goj, R., Jas, M., Brooks, T., Parkkonen, L., & Hämäläinen, M. (2013). MEG and EEG data analysis with MNE-Python. Frontiers in Neuroscience, 7, 267. https://doi.org/10.3389/fnins.2013.00267
Handwerker, D. A., Gonzalez-Castillo, J., D'Esposito, M., & Bandettini, P. A. (2012). The continuing challenge of understanding and modeling hemodynamic variation in fMRI. NeuroImage, 62(2), 1017–1023. https://doi.org/10.1016/j.neuroimage.2012.02.015
Iannetti, G. D., Niazy, R. K., Wise, R. G., Jezzard, P., Brooks, J. C. W., Zambreanu, L., Vennart, W., Matthews, P. M., & Tracey, I. (2005). Simultaneous recording of laser-evoked brain potentials and continuous, high-field functional magnetic resonance imaging in humans. NeuroImage, 28(3), 708–719. https://doi.org/10.1016/j.neuroimage.2005.06.060
Katan, M., & Luft, A. (2018). Global burden of stroke. Seminars in Neurology, 38(2), 208–211. https://doi.org/10.1055/s-0038-1649503
Keynan, J. N., Cohen, A., Jackont, G., Green, N., Goldway, N., Davidov, A., Meir-Hasson, Y., Raz, G., Intrator, N., Fruchter, E., Ginat, K., Laska, E., Cavazza, M., & Hendler, T. (2019). Author correction: Electrical fingerprint of the amygdala guides neurofeedback training for stress resilience. Nature Human Behaviour, 3(2), 194. https://doi.org/10.1038/s41562-019-0534-5
Keynan, J. N., Meir-Hasson, Y., Gilam, G., Cohen, A., Jackont, G., Kinreich, S., Ikar, L., Or-Borichev, A., Etkin, A., Gyurak, A., Klovatch, I., Intrator, N., & Hendler, T. (2016). Limbic activity modulation guided by functional magnetic resonance imaging-inspired electroencephalography improves implicit emotion regulation. Biological Psychiatry, 80(6), 490–496. https://doi.org/10.1016/j.biopsych.2015.12.024
Kilner, J. M., Mattout, J., Henson, R., & Friston, K. J. (2005). Hemodynamic correlates of EEG: A heuristic. NeuroImage, 28(1), 280–286. https://doi.org/10.1016/j.neuroimage.2005.06.008
Laufs, H., Krakow, K., Sterzer, P., Eger, E., Beyerle, A., Salek-Haddadi, A., & Kleinschmidt, A. (2003). Electroencephalographic signatures of attentional and cognitive default modes in spontaneous brain activity fluctuations at rest. Proceedings of the National Academy of Sciences of the United States of America, 100(19), 11053–11058. https://doi.org/10.1073/pnas.1831638100
Logothetis, N. K. (2002). The neural basis of the blood-oxygen-level-dependent functional magnetic resonance imaging signal. Philosophical Transactions of the Royal Society of London. Series B, Biological Sciences, 357(1424), 1003–1037. https://doi.org/10.1098/rstb.2002.1114
Mantini, D., Perrucci, M. G., Del Gratta, C., Romani, G. L., & Corbetta, M. (2007). Electrophysiological signatures of resting state networks in the human brain. Proceedings of the National Academy of Sciences of the United States of America, 104(32), 13170–13175. https://doi.org/10.1073/pnas.0700668104
Marecek, R., Lamos, M., Mikl, M., Barton, M., Fajkus, J., Rektor, I., & Brazdil, M. (2016). What can be found in scalp EEG spectrum beyond common frequency bands. EEG-fMRI study. Journal of Neural Engineering, 13(4), 046026. https://doi.org/10.1088/1741-2560/13/4/046026
Meir-Hasson, Y., Kinreich, S., Podlipsky, I., Hendler, T., & Intrator, N. (2014). An EEG Finger-Print of fMRI deep regional activation. NeuroImage, 102(Part 1), 128–141. https://doi.org/10.1016/j.neuroimage.2013.11.004
Meir-Hasson, Y., Keynan, J. N., Kinreich, S., Jackont, G., Cohen, A., Podlipsky-Klovatch, I., Hendler, T., & Intrator, N. (2016). One-class FMRI-inspired EEG model for self-regulation training. PLoS ONE, 11(5), e0154968. https://doi.org/10.1371/journal.pone.0154968
Moosmann, M., Schönfelder, V. H., Specht, K., Scheeringa, R., Nordby, H., & Hugdahl, K. (2009). Realignment parameter-informed artefact correction for simultaneous EEG–fMRI recordings. NeuroImage, 45(4), 1144–1150. https://doi.org/10.1016/j.neuroimage.2009.01.024
Murta, T., Chaudhary, U. J., Tierney, T. M., Dias, A., Leite, M., Carmichael, D. W., Figueiredo, P., & Lemieux, L. (2017). Phase-amplitude coupling and the BOLD signal: A simultaneous intracranial EEG (icEEG) - fMRI study in humans performing a finger-tapping task. NeuroImage, 146, 438–451. https://doi.org/10.1016/j.neuroimage.2016.08.036
Murta, T., Leite, M., Carmichael, D. W., Figueiredo, P., & Lemieux, L. (2015). Electrophysiological correlates of the BOLD signal for EEG-informed fMRI. Human Brain Mapping, 36(1), 391–414. https://doi.org/10.1002/hbm.22623
Niazy, R. K., Beckmann, C. F., Iannetti, G. D., Brady, J. M., & Smith, S. M. (2005). Removal of FMRI environment artifacts from EEG data using optimal basis sets. NeuroImage, 28(3), 720–737. https://doi.org/10.1016/j.neuroimage.2005.06.067
Ogawa, S., Lee, T. M., Kay, A. R., & Tank, D. W. (1990). Brain magnetic resonance imaging with contrast dependent on blood oxygenation. Proceedings of the National Academy of Sciences of the United States of America, 87(24), 9868–9872. https://doi.org/10.1073/pnas.87.24.9868
Pion-Tonachini, L., Kreutz-Delgado, K., & Makeig, S. (2019). ICLabel: An automated electroencephalographic independent component classifier, dataset, and website. NeuroImage, 198, 181–197. https://doi.org/10.1016/j.neuroimage.2019.05.026
Rosa, M. J., Daunizeau, J., & Friston, K. J. (2010). EEG-fMRI integration: A critical review of biophysical modeling and data analysis approaches. Journal of Integrative Neuroscience, 9(4), 453–476. https://doi.org/10.1142/s0219635210002512
Swartz Center for Computational Neuroscience. (n.d.). Makoto’s preprocessing pipeline. https://sccn.ucsd.edu/wiki/Makoto%27s_preprocessing_pipeline
Smith, S. M., Fox, P. T., Miller, K. L., Glahn, D. C., Fox, P. M., Mackay, C. E., Filippini, N., Watkins, K. E., Toro, R., Laird, A. R., & Beckmann, C. F. (2009). Correspondence of the brain's functional architecture during activation and rest. Proceedings of the National Academy of Sciences of the United States of America, 106(31), 13040–13045. https://doi.org/10.1073/pnas.0905267106
Stockwell, G. (2007). Why use the S-Transform? In L. Rodino, B.-W. Schulze, & M. W. Wong (Eds.), Pseudo-differential operators: Partial differential equations and time-frequency analysis (pp. 279–309). Providence, RI: American Mathematical Society.
UCL Queen Square Institute of Neurology. (n.d.). Statistical Parametric Mapping. Software. The Wellcome Centre for Human Neuroimaging. https://www.fil.ion.ucl.ac.uk/spm/software/
University of Oregon. (n.d.). MRIConvert files. Lewis Center for Neuroimaging. https://lcni.uoregon.edu/download/mriconvert
Wan, X., Riera, J., Iwata, K., Takahashi, M., Wakabayashi, T., & Kawashima, R. (2006). The neural basis of the hemodynamic response nonlinearity in human primary visual cortex: Implications for neurovascular coupling mechanism. NeuroImage, 32(2), 616–625. https://doi.org/10.1016/j.neuroimage.2006.03.040
Wei, C.-S., Lin, Y.-P., Wang, Y.-T., Lin, C.-T., & Jung, T.-P. (2018). A subject-transfer framework for obviating inter- and intra-subject variability in EEG-based drowsiness detection. NeuroImage, 174, 407–419. https://doi.org/10.1016/j.neuroimage.2018.03.032
Yin, S., Liu, Y., & Ding, M. (2016). Amplitude of sensorimotor Mu rhythm is correlated with BOLD from multiple brain regions: A simultaneous EEG-fMRI study. Frontiers in Human Neuroscience, 10, 364. https://doi.org/10.3389/fnhum.2016.00364
Downloads
Published
Issue
Section
License
Authors who publish with this journal agree to the following terms:- Authors retain copyright and grant the journal right of first publication with the work simultaneously licensed under a Creative Commons Attribution License (CC-BY) that allows others to share the work with an acknowledgement of the work's authorship and initial publication in this journal.
- Authors are able to enter into separate, additional contractual arrangements for the non-exclusive distribution of the journal's published version of the work (e.g., post it to an institutional repository or publish it in a book), with an acknowledgement of its initial publication in this journal.
- Authors are permitted and encouraged to post their work online (e.g., in institutional repositories or on their website) prior to and during the submission process, as it can lead to productive exchanges, as well as earlier and greater citation of published work (See The Effect of Open Access).
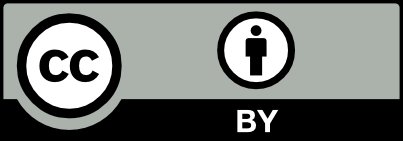